Imagine a factory where machines not only perform tasks but also anticipate maintenance needs, optimize production schedules and ensure quality, all autonomously. This is how AI is transforming industrial automation. For instance, Amazon has integrated advanced AI-driven robotics across its warehouses, deploying over 750,000 mobile robots and tens of thousands of robotic arms to enhance efficiency and reduce costs. These robots handle tasks ranging from heavy lifting to intricate package sorting, leading to a 25% reduction in order fulfillment costs and projected annual savings of $10 billion by 2030. Such advancements exemplify how AI is revolutionizing industrial processes, making operations more efficient, cost-effective, and adaptable to the demands of modern markets.
This blog aims to give you a deeper understanding of the role of AI in Industrial Automation through top AI solutions implemented by industries today.
Why Should You Read from Us?
As one of the leading Artificial Intelligence companies in India, Softlabs Group brings deep domain expertise and real-world implementation capabilities.
- 20+ Years of Expertise: Decades of experience in IT, delivering deep industry insights.
- Research-Backed Solutions: Our analysis is grounded in credible, thorough research.
- Tech Pioneers: Early adopters of Blockchain, AI/ML, and IoT, staying ahead of trends.
- Custom Solutions: Experts in crafting tailored software, IT consulting, and staff augmentation.
- Certified Excellence: ISO-certified, with multiple Clutch awards for quality and innovation.
The AI Advantage: Evolving from Analytics to Intelligence
Traditional data analytics helped industries make informed decisions, but it came with limitations — high infrastructure costs, time-consuming data processing, and limited adaptability. Most companies only saw ROI increases in the range of 10–20% despite heavy investment in legacy analytics systems.
But with the rise of Large Language Models (LLMs) and broader AI technologies, industrial automation has entered a new era.
Tools like GPT-4 and custom AI models now plug directly into existing workflows — from operations and customer service to safety and logistics — enabling companies to respond faster, automate smarter, and scale insights effortlessly.
From Limited Gains to Scalable Returns
📉 Traditional Analytics | 🚀 AI & LLM-Driven Automation |
ROI capped at ~10–20% | ROI rising to 230% + annually |
High infra and talent cost | Lightweight APIs and model-as-a-service options |
Static dashboards, reactive alerts | Predictive systems, real-time decisions |
Manual feature engineering | Self-learning models with contextual reasoning |
Example: In recent studies, companies using LLM-based automation reported up to 22.6% productivity boosts and 15.2% cost savings by reducing manual overheads and improving decision-making speed
AI Techniques Powering Industrial Transformation
Here’s a breakdown of core AI methods and where they shine on the factory floor:
🧠 AI Technique | 🏭 Industrial Use Case |
Supervised Learning | Quality assurance, cycle time prediction |
Unsupervised Learning | Fault detection, clustering sensor behavior |
Reinforcement Learning | Robotic path optimization, conveyor load balancing |
Computer Vision | PPE compliance, defect tracking, process automation |
Natural Language Processing (NLP) | Voice-controlled machines, maintenance ticketing, log parsing |
These aren’t just academic ideas — they’re already running in smart factories. Our own solutions, like real-time PPE detection and cover load monitoring, leverage a blend of vision systems and supervised models to boost safety and compliance in heavy industries. Even if having these in hand, let’s also check what exactly is blocking AI in industrial automation.
What’s Blocking AI in Industrial Automation?
AI holds great promise, but anyone who’s tried to implement it on the factory floor knows it’s not as simple as flipping a switch. Below are the real-world challenges that slow things down — and how industries are finding ways to deal with them, step by step.
1. Data Quality and Availability
AI relies on data — not just any data, but clean, well-organized, and consistent data. But most industries still have information scattered across spreadsheets, machines, old systems, and handwritten logs. This makes it hard for AI to learn or make reliable decisions.
Solution:
Start by figuring out where your most important data lives — maybe it’s in machine logs, ERP systems, or sensors. Then, pick one area (like energy use or machine uptime) and focus on cleaning and organizing that data first. You don’t need a full-blown overhaul — just a clear plan and a small, focused start. That’s often enough to launch a working model and prove value early.
2. Integration with Legacy Systems
Old equipment is everywhere. It wasn’t built for AI or even for sharing data easily. Replacing everything isn’t realistic, especially in traditional factories.
Solution:
Instead of tossing out old systems, companies are plugging in lightweight tools that can “listen” to those older machines and convert their signals into useful info. These are like translators — helping your old setup speak the same language as your new AI tools. It’s a smart workaround that keeps operations running while adding intelligence on top.
3. Workforce Resistance and Skill Gaps
Many workers feel like AI is being “done to them,” not with them. There’s fear of losing jobs, and confusion about how the new tools work.
Solution:
Involve your team from the start. Show them how AI can help reduce repetitive work, not taking away their roles. One smart move we’ve seen: companies ask teams to identify the biggest time-wasters or headaches in their day, then build AI tools to solve exactly those problems. When people see AI working for them, adoption happens naturally.
4. Data Privacy and Security Concerns
Feeding sensitive factory or production data into AI tools — especially cloud-based ones — can raise eyebrows about who has access and what happens if it leaks.
Solution:
Keep things simple: control who can see what, and use secure storage or tools that let you keep data on-site if needed. Being open about your data practices and setting clear access rules goes a long way in building trust with your team and leadership.
5. Accuracy and Bias in AI Models
Sometimes the AI gets it wrong. Maybe it misses a problem or raises a false alarm. This is especially frustrating when teams are already skeptical about using it.
Solution:
Don’t treat AI like a crystal ball. Use it as an assistant. Let it spot patterns, but keep people in the loop to make the final call — especially in areas like safety or quality. Over time, with feedback, the system learns and improves.
6. Ethical and Trust Issues
If an AI tool flags an issue or makes a recommendation, the natural question is: “Why?” If people don’t understand the answer, they’re less likely to trust or use it.
Solution:
Make sure the system can show a simple explanation for what it’s doing — even if it’s just “this reading is far above the usual range” or “this happened three times last month too.” You don’t need full transparency, just enough clarity to help people make a judgment call.
AI in Industrial Automation is powerful — but it’s not magic. It requires patience, small wins, and real involvement from the people who keep things running. Tackle the real blockers with simple, intentional actions, and the rest starts to fall into place.
If you are struggling with any of the above challenges, connect with us right away!
Innovative AI Solutions Transforming Industrial Automation
Picture this; a production engineer oversees a critical assembly line when a machine begins to vibrate abnormally — a subtle but crucial early sign of mechanical failure. In traditional setups, such signs often go unnoticed or are addressed too late during routine checks, resulting in costly downtime. Enter AI-powered predictive maintenance. By continuously analyzing vibration, temperature, and acoustic signals from embedded accelerometer and thermal sensors, the AI model flags abnormalities before they escalate. The technician receives an automated alert, enabling timely intervention that keeps production uninterrupted and repair costs minimal. This is just one example. Below are several AI-powered innovations built not just to automate but to relieve pressure from overstretched teams, reduce human error, and eliminate costly blind spots in industrial workflows.
1. AI-Powered Inventory Tracking Systems
Warehouse managers often struggle with inaccurate stock counts, unexpected shortages, or inefficient space usage. AI inventory systems now integrate RFID tags, IoT sensors, and computer vision to monitor stock levels in real-time. These systems not only detect exact item counts but analyze usage trends and reorder cycles using historical data. For example, if a particular component shows erratic demand due to seasonal spikes, the AI predicts this and flags it well in advance. This frees up supervisors from constant manual audits and prevents last-minute procurement chaos that can stall entire production lines. Here’s a quick snippet
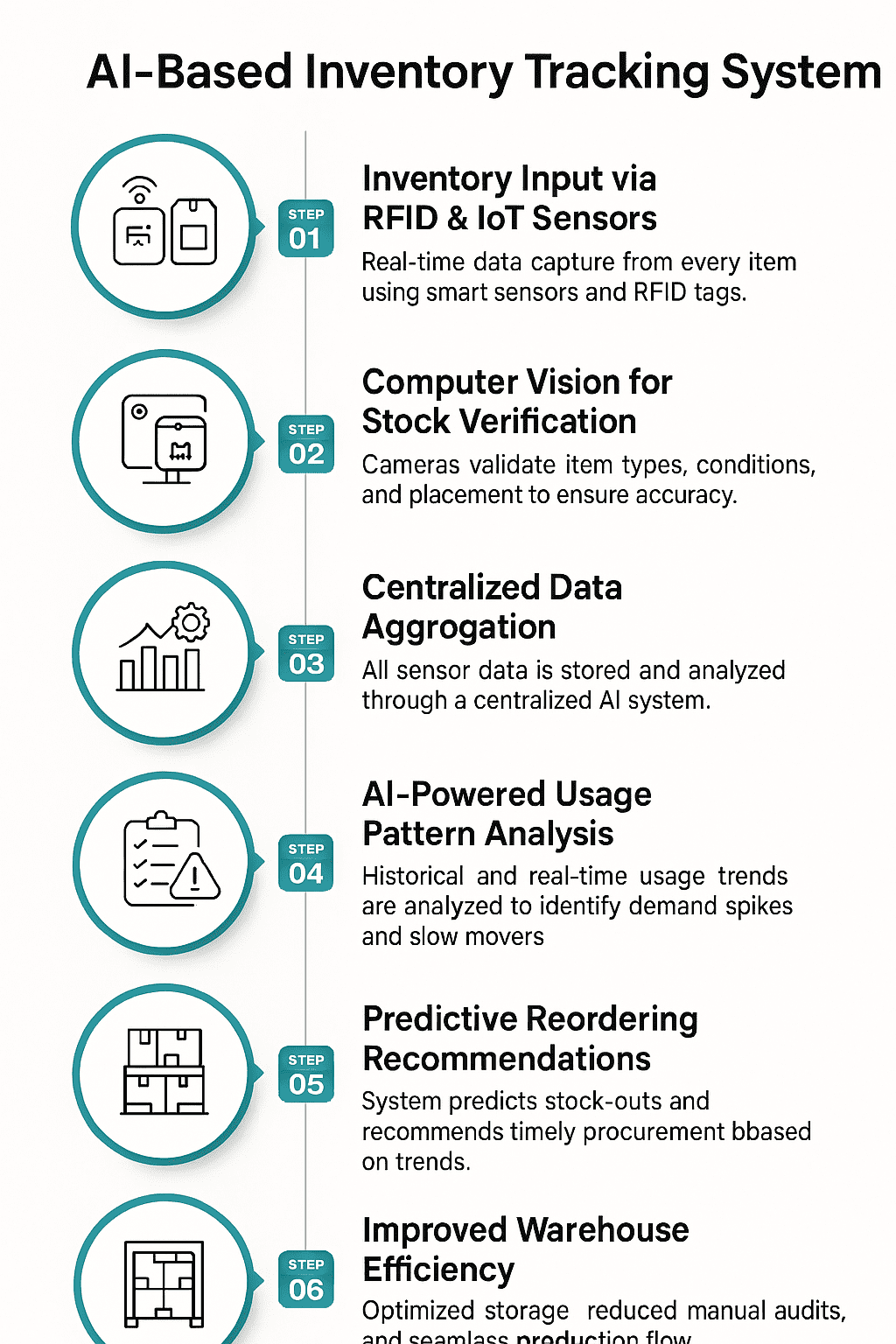
2. Automated Personal Protective Equipment (PPE) Detection
Safety managers in industries like oil & gas or mining know the stress of enforcing compliance. AI-enabled CCTV systems powered by object detection models (e.g., YOLO, SSD) now scan live footage to verify if workers are wearing mandated PPE like helmets, reflective jackets, or safety glasses. Instead of relying on periodic manual checks or end-of-shift reports, supervisors receive real-time alerts the moment non-compliance is detected. This not only closes the enforcement gap but builds a proactive safety culture without increasing manpower.
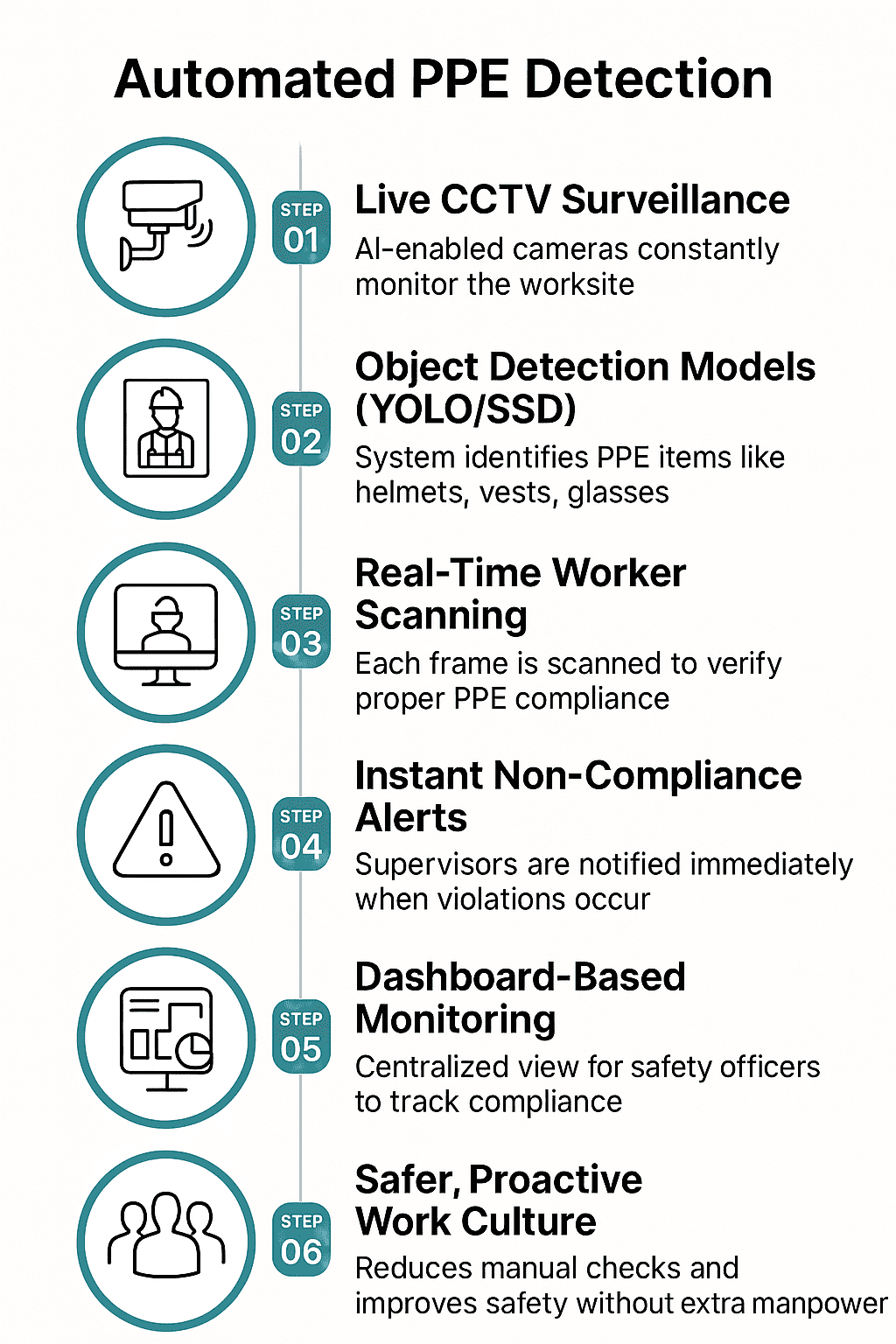
3. AI-Driven Production Tracking
Production managers often juggle between tracking KPIs, minimizing bottlenecks, and staying on schedule. With AI-based tracking systems, edge devices capture throughput data, cycle times, and machine idle durations across every station. A centralized dashboard powered by real-time analytics highlights deviations — say, if a robotic arm starts lagging due to friction buildup or sensor miscalibration. Instead of discovering this post-shift, the system triggers immediate intervention, helping managers stay ahead of inefficiencies and meet throughput targets with confidence.
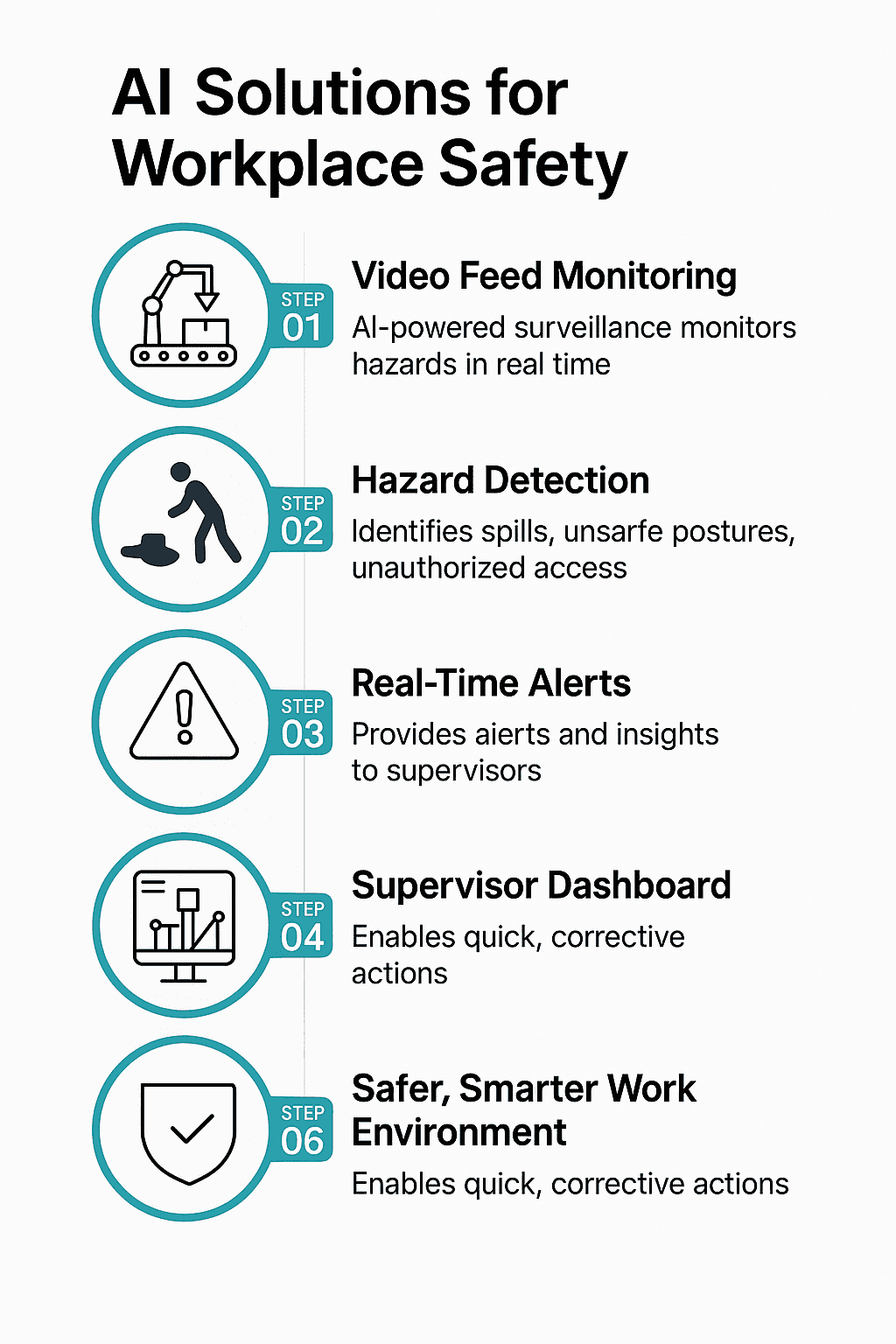
For logistics and operations heads, managing hundreds of vehicles means constantly reacting to breakdowns, delays, and rising fuel costs. AI fleet platforms ingest telematics data, including engine performance, GPS routes, braking patterns, and fuel efficiency. Predictive models recommend optimal maintenance schedules and dynamic routing. For instance, if a delivery truck shows repeated harsh braking, the system links this to increased tire wear and preschedules a service. This shift from reactive to proactive fleet management improves vehicle longevity, on-time deliveries, and operational cost savings.
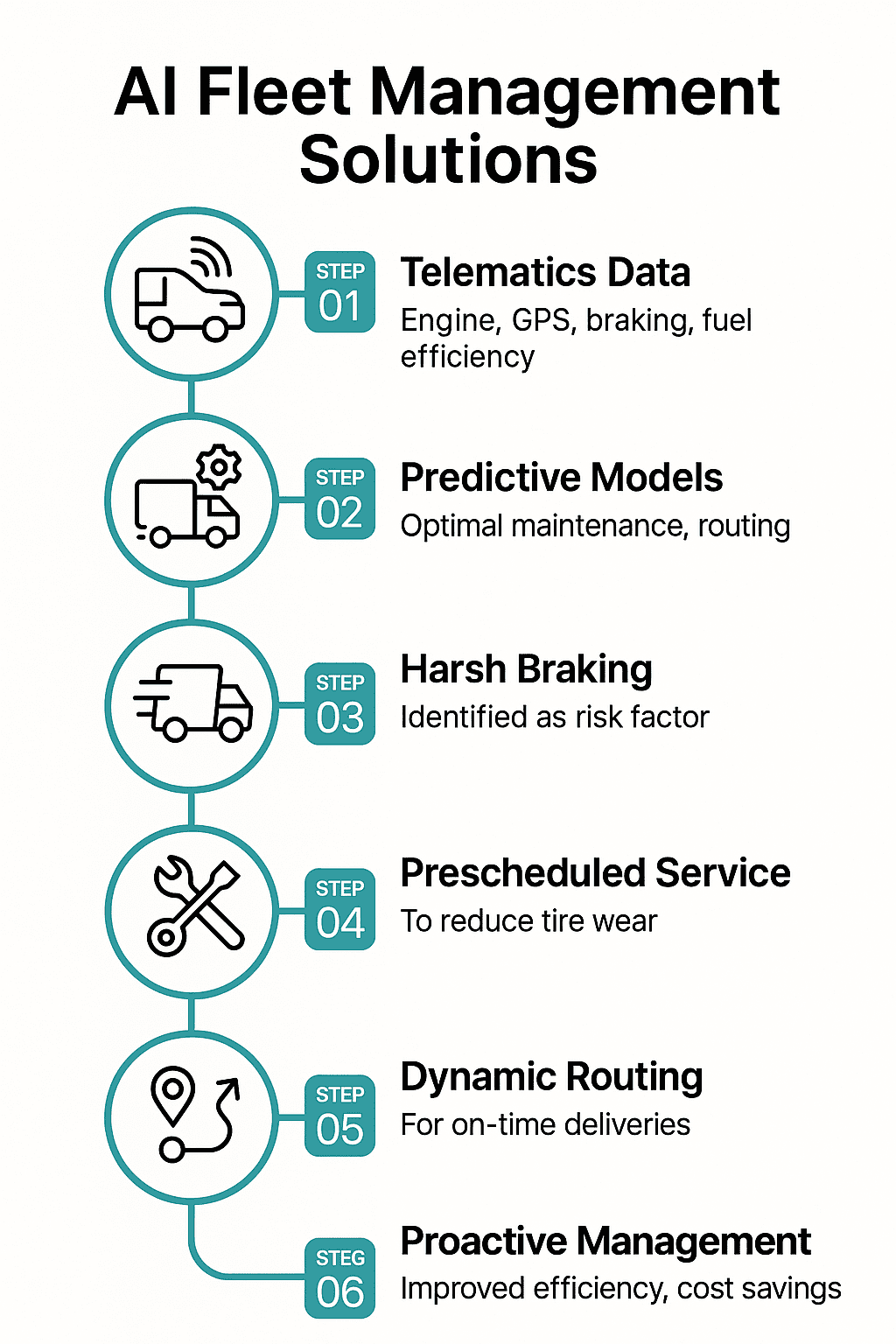
5. AI Solutions for Workplace Safety
Plant supervisors often walk a tightrope, ensuring production speed without compromising safety. AI-powered surveillance systems now use video analytics and anomaly detection algorithms to monitor shop floor hazards in real-time. Whether it’s spotting a spill near electrical equipment, recognizing unsafe body posture near conveyors, or detecting unauthorized access to high-risk zones, the system acts like an ever-vigilant assistant. Instead of relying on scattered manual logs, supervisors get consolidated insights and alerts, allowing for quick, corrective decisions that keep teams safe and operations smooth.
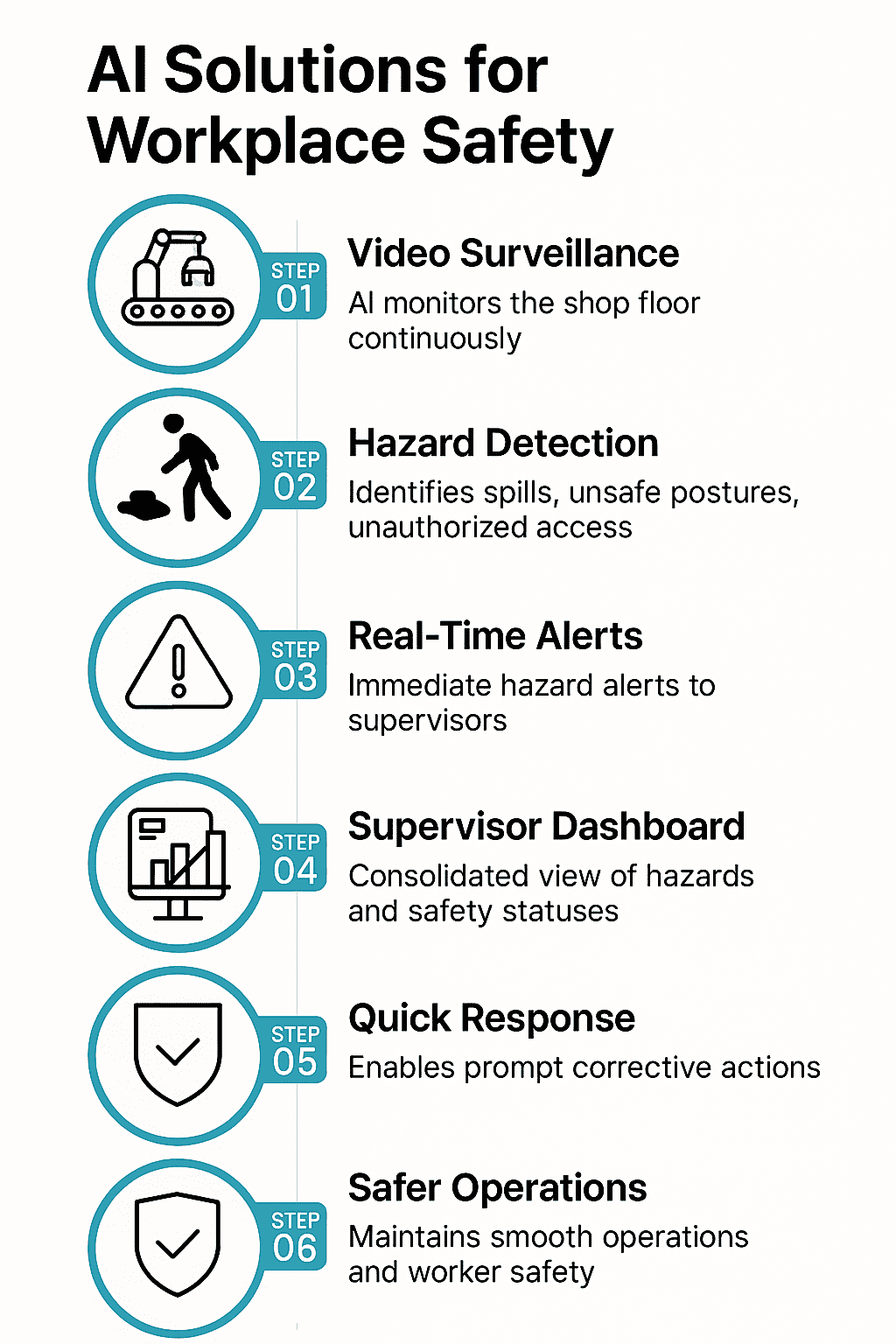
6. Worker Safety and Vibration Monitoring
In sectors like construction and heavy manufacturing, long-term exposure to tool vibrations is a silent threat. Operators using jackhammers or grinders often suffer from HAVS (Hand-Arm Vibration Syndrome), yet rarely know when they’re exceeding exposure limits. Wearable AI devices with triaxial accelerometers track vibration signatures in real-time, distinguishing tool vibration from normal body movement. When thresholds based on ISO 5349 standards are approached, both the worker and supervisor get instant notifications via a paired dashboard. If a sudden shock or fall is detected, the system even triggers emergency protocols. This not only minimizes health risks but also empowers workers with visibility into their safety.
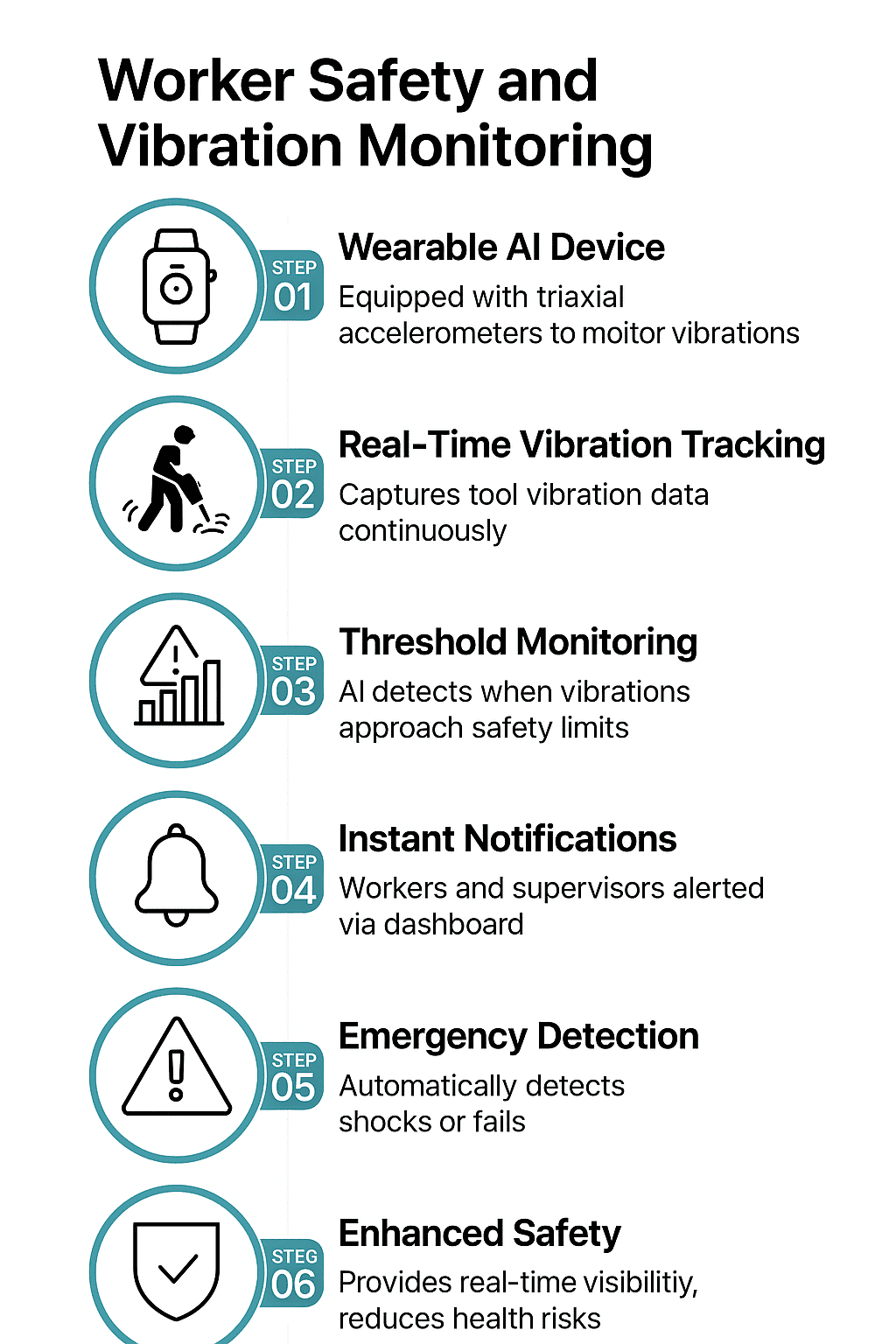
7. AI-Powered Quality Inspection in Manufacturing
Manufacturers often grapple with maintaining consistent product quality, which can lead to increased waste and customer dissatisfaction. Implementing AI-driven visual inspection systems enables real-time detection of defects on production lines. These systems utilize machine learning algorithms to analyze images from high-resolution cameras, identifying inconsistencies that may be missed by the human eye. For instance, a leading electronics manufacturer integrated an AI-based inspection system, resulting in a significant reduction in defect rates and improved overall product quality. Automate
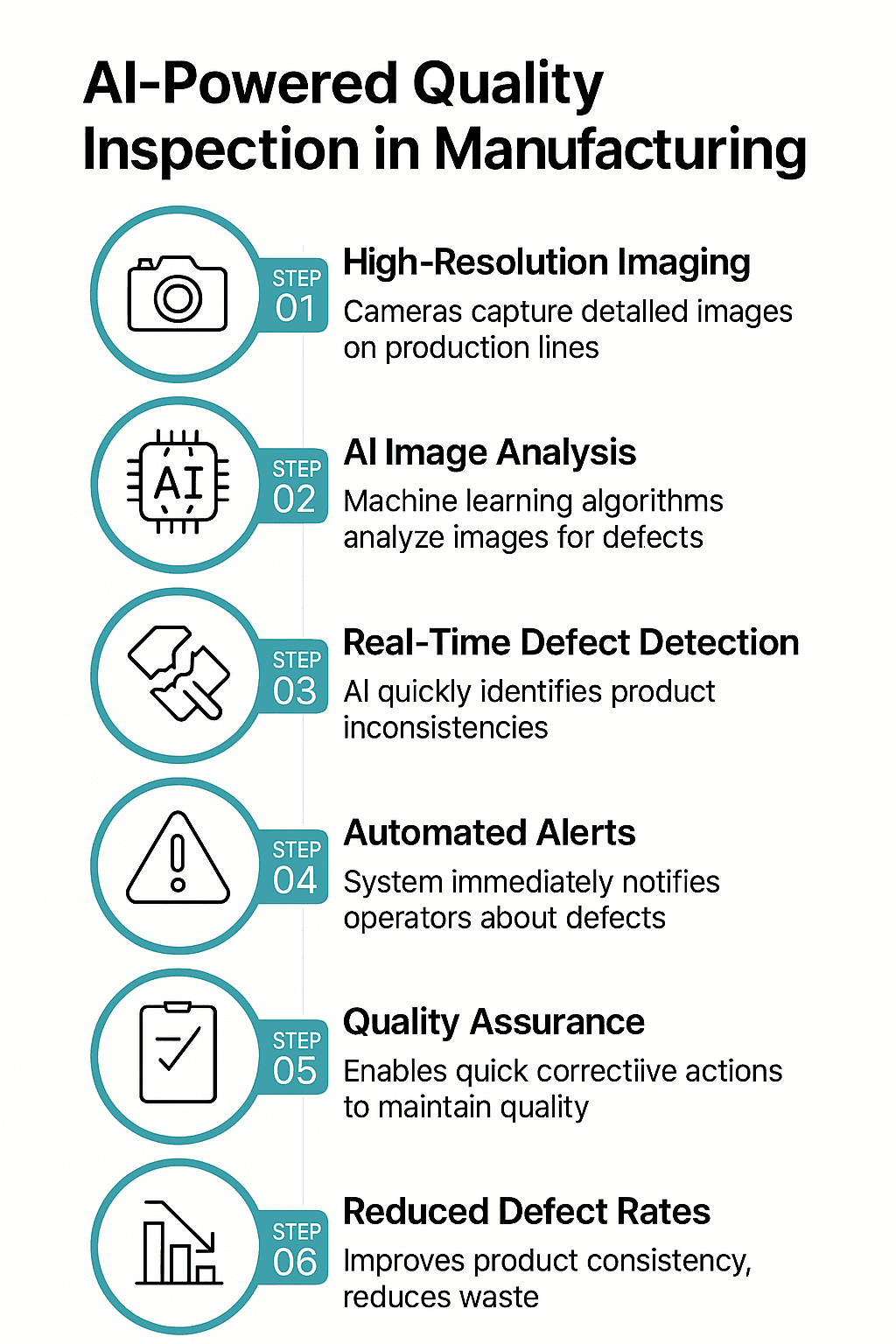
8. AI-Driven Predictive Maintenance in Food Processing
Equipment failures in food processing can lead to costly downtime and product spoilage. By deploying AI-driven predictive maintenance systems, companies can monitor machinery health in real-time. These systems analyze data from sensors to predict potential failures before they occur. For example, PepsiCo’s Frito-Lay plants implemented such a system, achieving a 4,000-hour increase in production capacity by minimizing unplanned downtime. AIMultiple
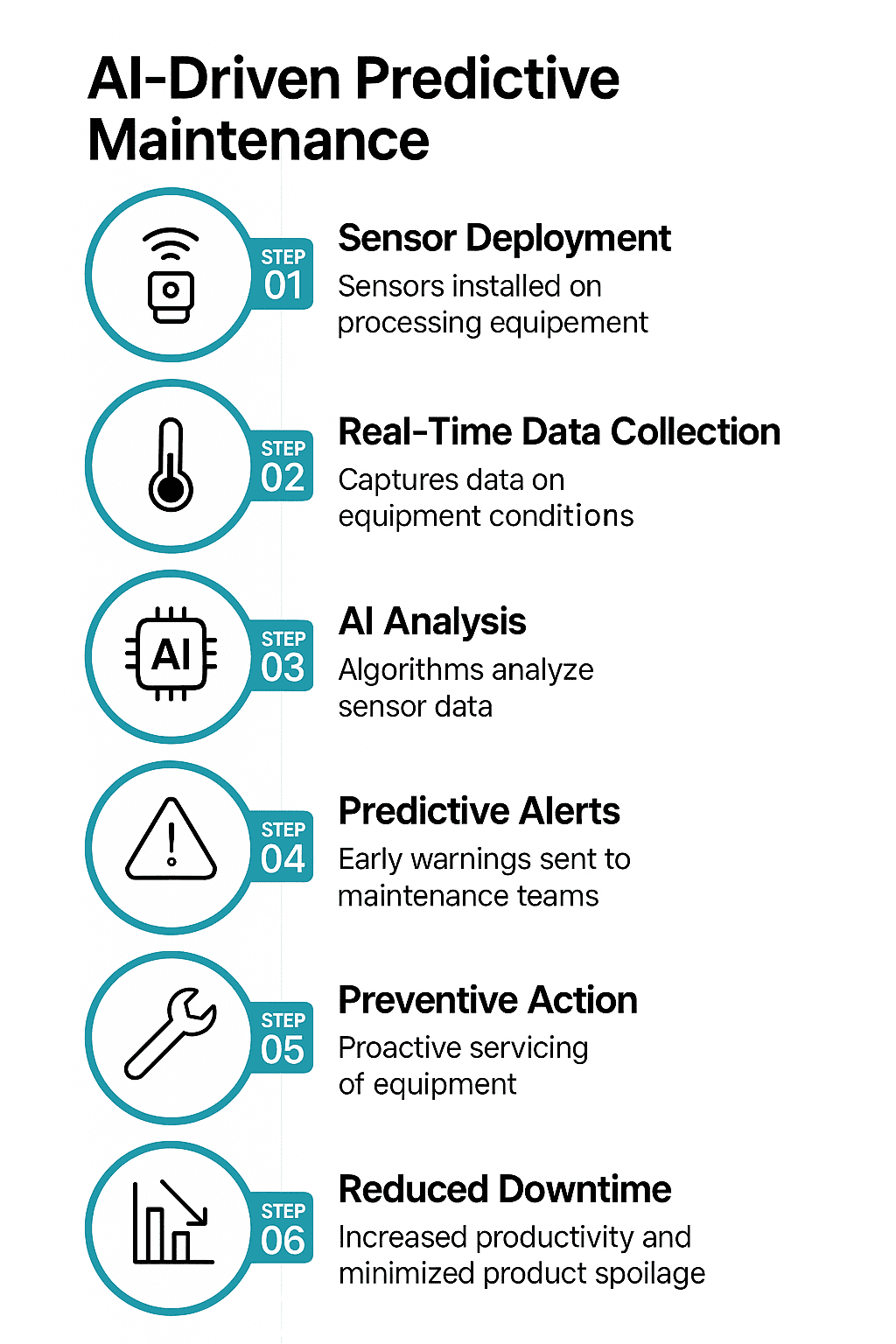
9. AI-Enhanced Production Scheduling
Coordinating complex production schedules to meet demand while optimizing resource utilization is a common challenge in manufacturing. AI-enhanced scheduling systems analyze various factors, including machine availability, labor resources, and supply chain constraints, to generate optimal production plans. Honeywell, for instance, leverages AI to optimize production scheduling in their factories, leading to reduced lead times and improved customer satisfaction. AIMultipleMSR Cosmos
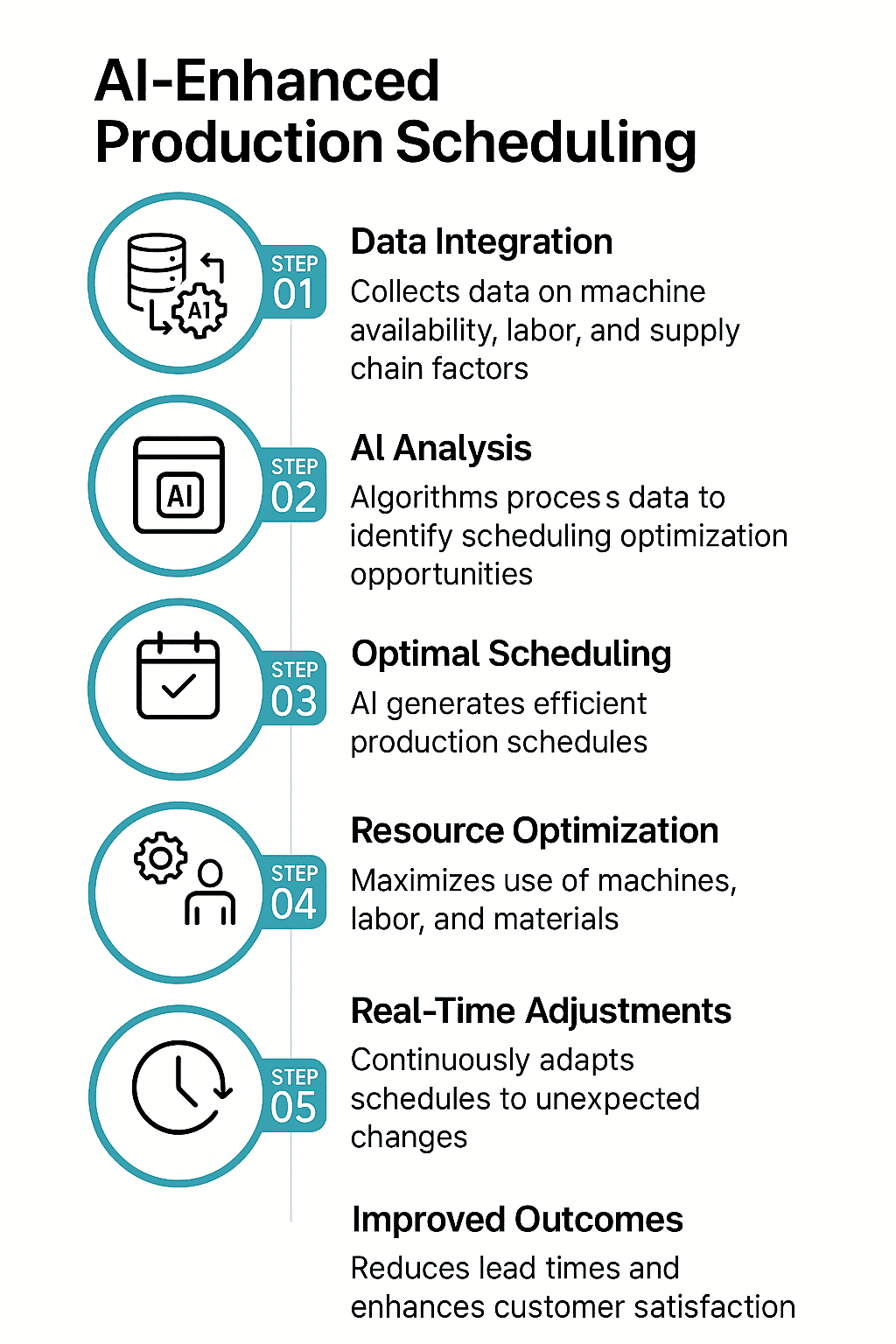
10. AI-Powered Route Optimization in Logistics
Logistics companies face challenges in determining the most efficient delivery routes, which impact fuel costs and delivery times. AI-powered route optimization tools analyze real-time traffic, weather, and road conditions to recommend efficient routes. UPS’s ORION system, for example, has cut delivery miles and saved over $400 million annually through AI-driven route planning. Thoughtful
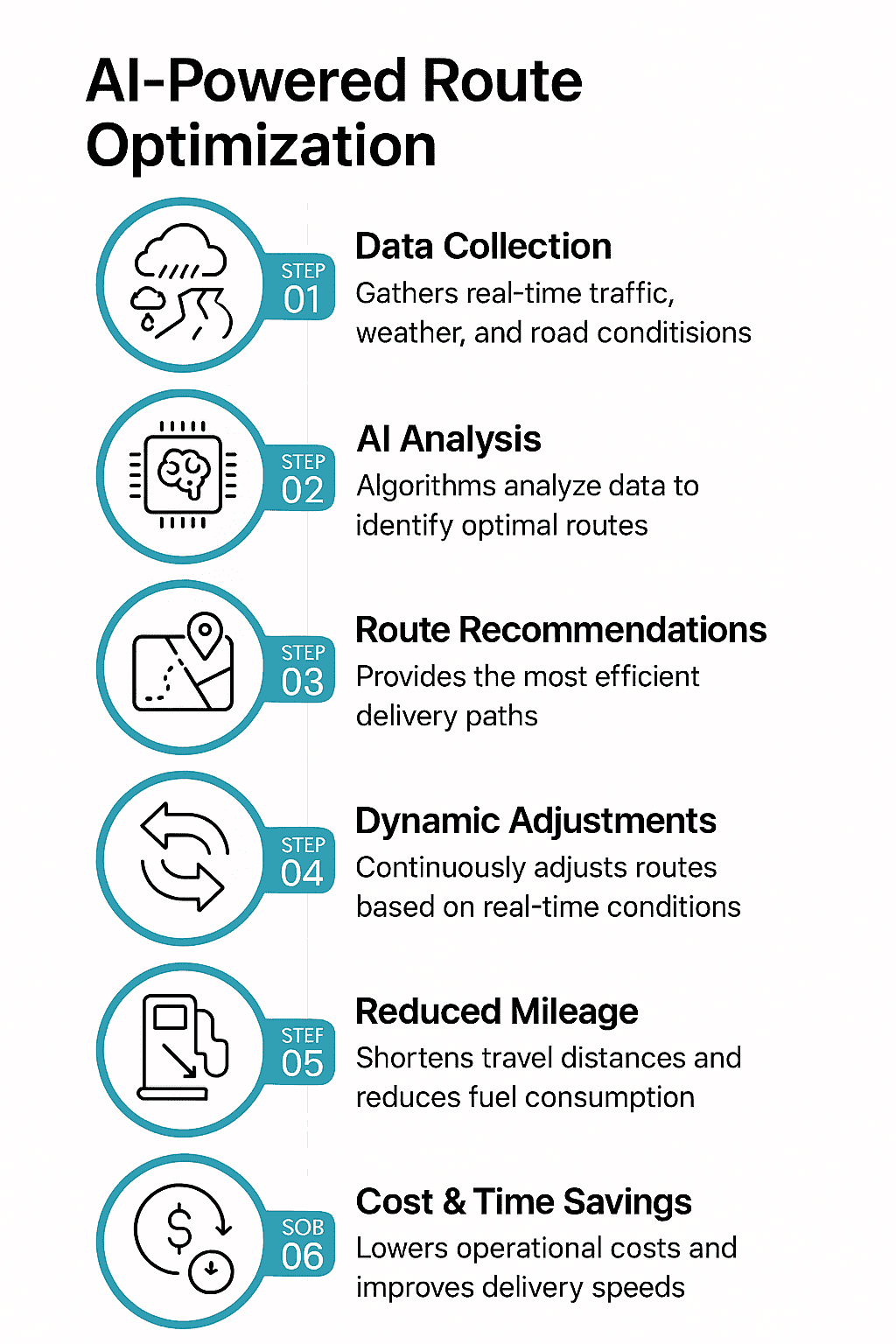
11 AI-Integrated Robotics for Warehouse Automation
Warehouses often struggle with labor-intensive tasks such as sorting and packing, which can be time-consuming and prone to errors. Integrating AI with robotics enables automated systems to learn and adapt to various tasks, improving efficiency and accuracy. For instance, Covariant has developed AI-powered robotic arms capable of handling diverse items, enhancing warehouse operations for companies like ABB and Knapp. Wikipedia
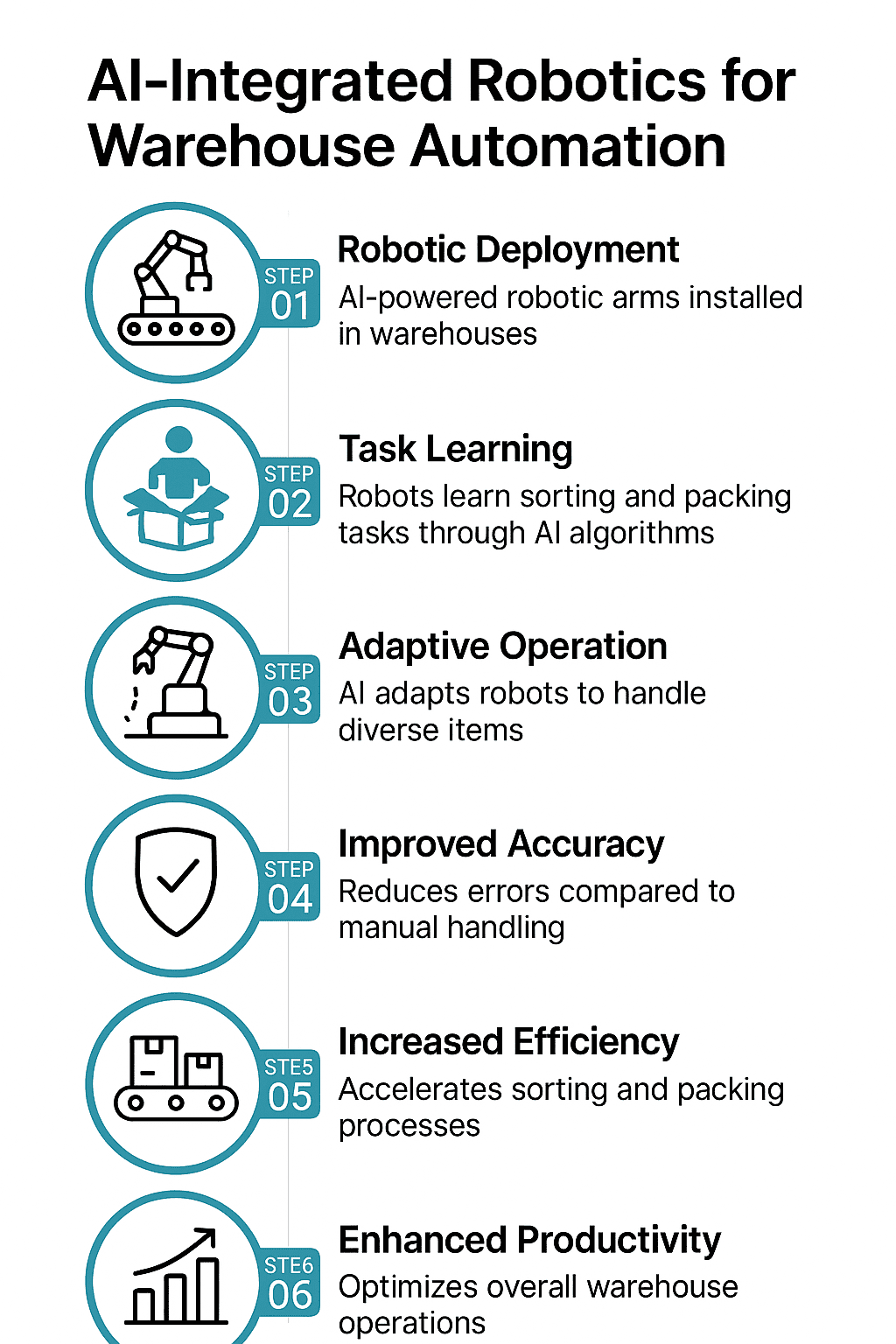
By embedding these AI solutions into their operations, industries aren’t just automating—they’re empowering plant teams, relieving mental bandwidth, and transforming reactive firefighting into strategic foresight. From frontline operators to decision-makers in the control room, AI is the silent partner reshaping industrial automation with intelligence and empathy.
Real-World AI Implementations in Industrial Automation
The integration of Artificial Intelligence (AI) in industrial automation has led to significant advancements across various sectors. Below are case studies illustrating how AI solutions have addressed critical challenges, the solutions implemented, and the measurable impacts achieved.
1. Enhancing Compliance and Safety in Material Transportation
Problem: A leading UK manufacturer in the construction industry faced challenges in ensuring compliance and safety during material transportation.
Solution: SoftLabs Group developed an AI-powered solution that monitors and analyzes transportation processes to enhance compliance and safety measures.
Impact:
- Achieved a 30% reduction in safety incidents related to material transport.
- Improved compliance with transportation regulations by 25%.
Check out the whole case study here!
2. Production delays and increased maintenance costs.
Solution: Festo implemented its AI-based predictive maintenance tool, Festo Automation Experience (AX), which analyzes machine data in real-time to predict and prevent equipment failures. Festo
Impact:
- Achieved significant cost savings per machine by reducing unexpected downtimes.
- Enhanced equipment reliability and operational efficiency.
Source: Festo Case Study
2. AI-Driven Quality Control in Electronics Manufacturing
Problem: An electronics manufacturer struggled with detecting defects during production, affecting product quality and customer satisfaction.
Solution: Foxconn partnered with Huawei to implement an AI-powered quality inspection system using Ascend AI, which automatically inspects smart PV controllers for defects such as incorrect silicone grease application and misattached nameplates. AI in Manufacturing is transforming traditional assembly lines by detecting errors earlier and ensuring product consistency. Huawei Enterprise
Impact:
- Inspected over 6,000 devices monthly with an accuracy exceeding 99%. Huawei Enterprise
- Significantly reduced defect escape rates and operational costs. Conformance1
Source: Huawei Case Study
3. AI Visual Inspection in Electronics Manufacturing
Problem: Might Electronics faced challenges in accurately measuring cycle times and identifying production bottlenecks due to reliance on manual inspections. Advantech
Solution: The company introduced the PowerArena AI visual inspection program, deploying cameras at each workstation to collect images and send them to the Advantech SKY-6400 server for AI analysis. This system enabled instant calculation of cycle times and identification of inefficiencies.
Impact:
- Improved accuracy in cycle time measurement.
- Enhanced identification and resolution of production bottlenecks.
Source: Advantech Case Study
Latest AI Trends for Industrial Automation
The industrial automation landscape is rapidly evolving, with Artificial Intelligence (AI) at the forefront of this transformation. Below are some of the most impactful AI trends currently reshaping the industry:
1. AI-Powered Digital Twins
Digital twins are virtual replicas of physical assets, processes, or systems that enable real-time monitoring, simulation, and optimization. By integrating AI, these digital models can predict equipment failures, optimize production schedules, and enhance overall operational efficiency. For instance, NVIDIA and Schneider Electric have partnered to create digital twins for AI data centers, aiming to improve power consumption and operational efficiency.
2. Generative AI for Design & Optimization
Generative AI algorithms assist in creating innovative designs by exploring numerous possibilities within specified constraints, leading to more efficient and sustainable solutions. In the manufacturing sector, companies like Autodesk have collaborated with Airbus to develop lighter and more efficient aircraft components using generative design, resulting in significant weight reduction and fuel efficiency.
3. Edge AI
Edge AI involves processing data locally on devices or machines, bringing computation closer to the data source. This approach reduces latency, enhances real-time decision-making, and improves data privacy. For example, STMicroelectronics has launched microcontrollers designed for edge AI applications, enabling devices to perform complex tasks like image and audio processing on-site without relying on centralized data centers.
4. Human-AI Collaboration
Collaborative robots, or “cobots,” exemplify the synergy between humans and AI, working alongside human workers to enhance productivity and safety. Companies like Universal Robots have developed cobots that are easily programmable and can be integrated into existing production lines without extensive modifications, facilitating tasks such as assembly, packaging, and quality inspection.
5. Explainable AI (XAI)
As AI systems become more complex, the need for transparency and trust in AI-driven decisions has grown. Explainable AI (XAI) focuses on making AI models more interpretable, allowing humans to understand and validate the decisions made by these systems. Industrial operations today leverage various types of AI, from narrow AI in visual inspection to advanced contextual models for strategic planning. This is particularly crucial in industries where safety and compliance are paramount. Efforts are underway to develop methodologies that enhance the interpretability of AI models, thereby fostering trust and facilitating broader adoption in industrial settings. Source: LinkedIn
Embracing the Future of Industrial Automation
The future of industrial automation is being shaped by AI, paving the way for smart factories, autonomous logistics, and data-driven decision-making. Smart factories leverage AI to create highly adaptive environments where machines self-optimize processes, predict maintenance needs, and ensure product quality with minimal human intervention. Autonomous logistics is another game-changer, with AI streamlining supply chains, optimizing delivery routes, and ensuring faster, more reliable transportation of goods. These advancements aren’t just top AI use cases enhancing efficiency — they’re about creating more resilient and agile industrial ecosystems. Source: Morgan Stanley
As Industry 4.0 evolves, the road ahead points towards greater collaboration between humans and intelligent systems. AI is no longer a futuristic concept but a necessity for industries aiming to stay competitive. From reducing downtime to improving safety and sustainability, the integration of AI promises to redefine the very essence of industrial work. The question is no longer if industries should adopt AI, but how quickly they can embrace the change. As we stand on the cusp of this transformation, one can’t help but wonder: How far will AI push the boundaries of what’s possible in industrial automation?
Pingback: AI in Mechanical Engineering - News Directory 3