Summary:
In the realm of formulation development, the integration of artificial intelligence (AI) presents a powerful toolkit for scientists and researchers. The process begins with AI-driven optimization algorithms, particularly Bayesian optimization, to expedite the development of new formulations. This iterative approach, starting with minimal experimental data, progressively refines machine learning models, leading to more effective and efficient formulation outcomes.
For reformulation challenges, the same optimization algorithms are employed, but with a different focus—adapting existing formulations to incorporate new ingredients. Leveraging a larger dataset acquired during the development of the original formulation, this approach aims to maintain or enhance product performance and stability while minimizing the number of experiments required.
Furthermore, AI plays a crucial role in testing new formulation ideas through traditional machine learning methods. By building models from accumulated formulation data, scientists can predict the properties of hypothetical formulations, allowing for in silico validation before committing to physical experiments. This mitigates risks, saves time, and optimizes resources.
Despite differences in approaches, the common thread is the ability to harness AI to make data-driven decisions, whether in the creation of new formulations, the adaptation of existing ones, or the virtual testing of novel ideas. Each method addresses specific challenges in formulation development, contributing to a more efficient and cost-effective research and development process.
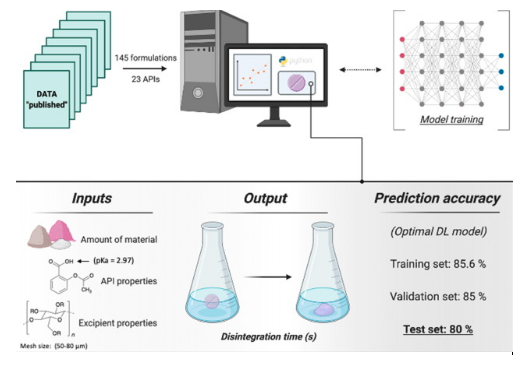
AI for Developing New Formulations:
- Conduct a small number of initial experiments (minimum of 2).
- Build a Gaussian process machine learning model using the experiment results, providing uncertainty with predictions.
- Employ Bayesian optimization to choose the next experiments by balancing exploitation (trusting the model) and exploration (running experiments with high uncertainty).
- Run proposed experiments, add results to the dataset, and iterate.
- Stop when a formulation meeting objectives is achieved or experiment budget is exhausted.
- Note: Bayesian optimization enhances efficiency by capitalizing on experiment results, and it’s effective even with minimal initial data.
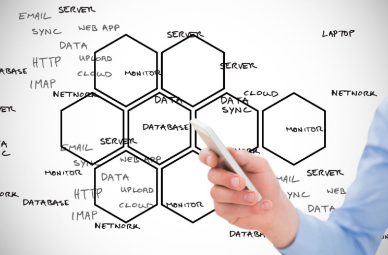
AI for Reformulation:
- Utilize AI-based optimization algorithms (e.g., Bayesian optimization) to replace raw materials in an existing formulation.
- Apply the same process as new formulation development but start with a larger dataset (existing formulation data).
- Objective: Maintain or improve the performance and stability of the original formulation.
AI to Test Formulation Ideas:
- Build machine learning models using accumulated formulation data.
- Predict properties of hypothetical formulations for in silico testing.
- Traditional machine learning approach without optimization.
- Validate formulation ideas before conducting physical experiments.
- Requires substantial data; recommended data points at least 5-10 times the number of inputs.
- Addresses the lack of diversity in datasets from human-designed experiments.
Key Advantages
- Bayesian optimization accelerates formulation development with minimal initial data.
- AI-based optimization for reformulation minimizes experiments needed for ingredient replacement.
- Traditional machine learning enables in silico testing of formulation ideas, validating concepts before physical testing.
- AI-designed experiments in optimization overcome limitations of datasets lacking diversity.
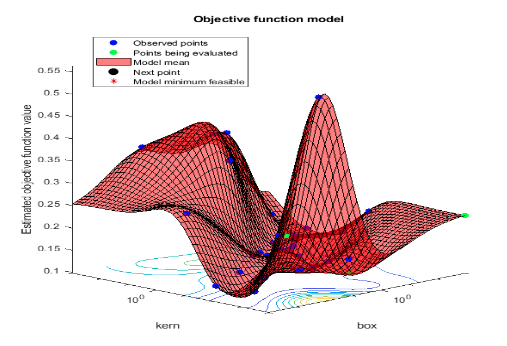
Considerations:
- Bayesian optimization becomes more powerful with each iteration, capitalizing on experiment results.
- Reformulation AI leverages existing formulation data for efficient adaptation to new ingredients.
- Traditional machine learning requires substantial data and may lack diversity in datasets.
Conclusion
The integration of AI in formulation development stands as a transformative force, offering agility and precision in navigating the complexities of creating and optimizing formulations. Bayesian optimization emerges as a key player, enabling accelerated progress with minimal initial data, a feature particularly advantageous in the early stages of development.
Reformulation, a common need in dynamic markets, benefits from the adaptability of AI algorithms, ensuring that changes to raw materials are made with a data-driven focus on maintaining or improving product characteristics. Traditional machine learning adds a layer of predictive capability, allowing scientists to assess formulation ideas virtually, thereby reducing the reliance on resource-intensive physical experimentation.